Applications of AI in hiring
AI technologies for hiring take on many forms and are used at different stages of the hiring process. A notable application of AI in hiring is for the sourcing and screening stages. In high-volume hiring, recruiters and hiring managers benefit greatly from AI tools that parse through the candidates in their ATS and narrow the hiring pool based on objective metrics, such as years of experience or relevant skills. When dealing with upwards of 250 or more applications for an opening, recruiting teams save countless hours by integrating algorithms into the screening and sourcing processes. Predictive technologies sort, analyze, and score resumés, allowing hiring managers to assess candidate competencies by leveraging both traditional and novel data. Outside of the hiring process itself, AI has many applications in the job search. AI is also used for job ad targeting by steering job ads towards desired candidates; other tools are used to identify passive candidates for recruitment. These digital sourcing applications to stay competitive, seeing as 87% of recruiters use LinkedIn to source and check candidates.
NLP and chatbots
Many organizations have adopted NLP, or natural language processing, into their candidate journey. Specifically, recruitment chatbots that walk candidates through their application in an intuitive and user-friendly way similar to chat services commonly used for customer interaction. Aside from walking candidates through the application process, chatbots can be used to schedule interviews and respond to candidate queries about the company. Not only do chatbots provide a positive candidate experience for applicants, but these tools also provide valuable data and insights about the hiring pool to employers through machine learning and database building.
Human bias
A commonly held belief about AI is that it is capable of removing unconscious human bias. On average, recruiters take about 6 seconds to scan a resumé. This is hardly enough time to form a holistic understanding of a candidate’s viability and leads recruiters to make snap judgements or write off potentially great candidates. Algorithms are able to capture more information than a human recruiter in less time. Furthermore, hiring managers are often influenced by personal experiences and biases that are not factors for AI. For example, people are generally susceptible to similarity attraction bias. This means that humans have an instinct to surround themselves with those they feel they have commonalities with. Many attributes can be factored into this, such as where a candidate went to school, or even their race, age, gender, and other characteristics that should not be determinants as to whether or not someone is hired. When it comes to these personal biases, AI is generally more objective and more efficient than people. However, it is not completely infallible.
How does AI perpetuate bias in hiring?
By virtue of being man made, no technology can be completely free from bias. While many hope that algorithms help decision-makers avoid their personal prejudices and biases, algorithms can amplify institutional and historical biases. An algorithm’s level of objectivity is correlated with the objectivity of the data it draws from and that data, in many cases, can be biased. Hiring is a multi-step process and rarely comes down to a single decision. Rather, a hire is the result of a series of sequential decisions that eventually establish the final outcome. Developing an understanding of algorithmic bias requires analyzing how predictive technologies function at each stage of the hiring process. Tools used earlier in the process are fundamentally different from those used later on and can impact the hiring process in different ways. Even tools that may initially seem similar may rely on completely different types or sets of data and can deliver predictions in distinct ways.
AI bias in sourcing
A recent study by the Harvard Business Review revealed underlying biases in targeted job ads on Facebook. One finding was that broadly targeted ads for supermarket cashier positions were shown to an audience that was 85% female. In another instance, jobs with taxi companies were shown to an audience that was 75% Black. This is a typical outcome when algorithms draw from data that is historically biased. When these inherent biases go unchecked by human intervention, this hurts the hiring pool and fails to provide an equitable experience for all candidates.
AI bias in screening and employee evaluation
Some selection tools incorporate machine learning to make predictions about which applicants will be most successful on the job. These predictions are based on metrics related to tenure, productivity, and performance, all mined through historical company data. If this performance data is compromised by sexism, racism, or other structural biases, this will be reflected in the AI functionality. Currently, the bar for evaluating the impartiality of these evaluation tools is quite low. While employers are obligated to analyze their assessment instruments for bias, as long as companies can prove that their selection tools serve a concrete business interest, its use can be justified even if it results in inequitable outcomes. Seeing as diverse organizations are 45% likelier than their counterparts to increase their market share, compromising on objectivity in AI-powered screening is not in business’ best interest.
Considerations for employers when using AI
A 2019 study from Delft University of Technology on the fairness of AI recruitment systems established several best practices for employers to continually monitor and assess their AI processes to ensure an equitable outcome for candidates. The efficiency and unbiased nature of AI is contingent upon its continued monitoring and modification by people. The resultant recommendations are as follows:
Justification: Does it make sense for an organization of a certain size with specific hiring needs to employ AI hiring tools, given the data requirements and the need for bias remediation?
Explanation: Does the AI tool explain its decisions and are those explanations made available to the recruiter and the applicant? If algorithmic information is proprietary, are counterfactual explanations taken into consideration?
Anticipation: Are mechanisms in place to report biased decisions and what are the recourse mechanisms in place?
Reflexiveness: Is the organization aware of its changing values and its reflection in the data it uses? How is data collected and which limitations become evident?
Inclusion: Do you think about diversity in your team and in the evaluation results?
Auditability: Is the training data publicly available or verified by a third party?
Final takeaways
When bias permeates AI recruitment tools, all parties are worse off. Candidates are not offered an equitable experience and employers miss out on valuable applicants and can fail to diversify their teams. AI may be effective at mitigating the knee-jerk unconscious biases that human recruiters perpetuate, but it is by no means completely impartial. Due to the fact that algorithms are man-made and often draw from inherently biased datasets, AI does not guarantee objective and fair outcomes. No matter how sophisticated, AI’s fallibility necessitates continued human input.
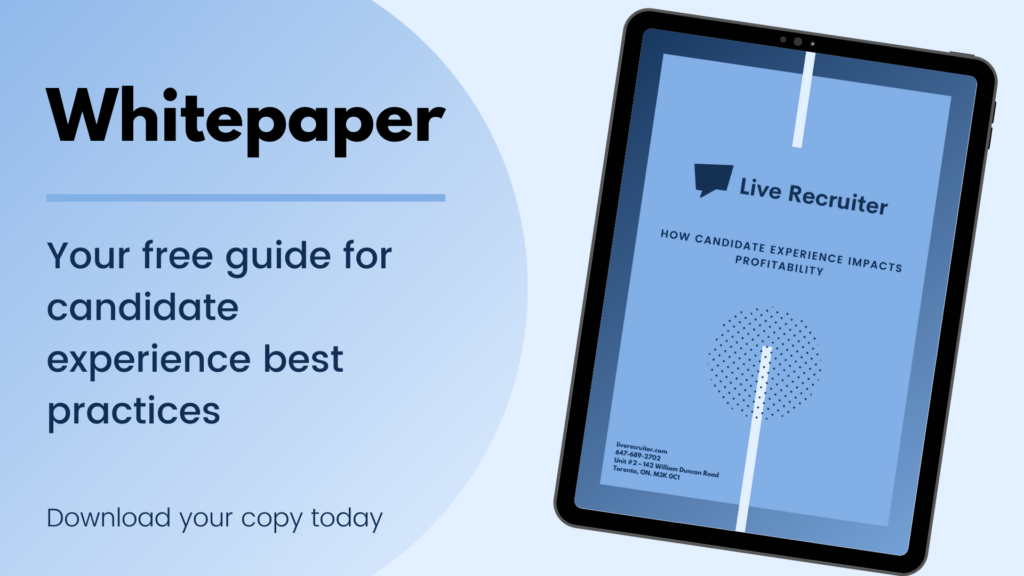